Best Predictor Variables Selection in Logistic Regression Modeling for Credit Card Payment Data
Abstract
Credit scoring is a method used to evaluate credit risk in terms of loan applications from debtors. This method is used to determine the provision of credit limit from the debtor based on the payment behavior performed by the debtor. In the process because we are dealing with very large data either the number of variables or the number of observations, then we do the workmanship techniques based on the principle of data mining. Where the selection of best predictor variables will be selected based on the value of information value greater than 10% obtained using unsupervised learning techniques and modeling techniques in credit scoring is by using logistic regression which is a supervised learning technique in data mining. From the result of data transformation, correlation and modeling examination with backward elmination of 23 predictor variables obtained 5 best predictor variables modeled to determine the behavior of payments made by credit card customers in Taiwan, namely; Historical payment of the month of April 2005, the amount of the previous payment in June 2005, the amount of the previous payment in July 2005, the previous payment amount in August 2005, and the amount of previous payment in September 2005. Then after the best predictor variable is obtained we do logistic regression modeling, With the value of KSnya 50.33% and 60.84% and the value of GINI coefficient 53.83% and 62.56%, then the model can be said reliable in separating the debtor included in the good debtor and bad debtor.
Keywords
Full Text:
PDF (Bahasa Indonesia)References
Amaranth, K.N. (2004).Statistical Methods in Consumer Credit Scoring. Cranes Software Internasional Ltd . Product Abalyst .
Davies, B. (2004). Database Systems 3rd Edition. Palgrave. Basingstoke. UK.
Hajarisman, N. (2009). Analisis Data Kategorik. Bandung: Program Studi Statistika, Universitas Islam Bandung.
Naeem S. (2006). Credit Risk Scorecards: Developing and Implementing Intelligent Credit Scoring. John Wiley & Sons.
Nguyen H. (2014). Default Predictors in Credit Scoring – Evidence from France’s Retail Banking Institution. Journal of Economic, 26, 1-20
DOI: http://dx.doi.org/10.29313/.v0i0.7738
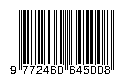