Perbandingan Estimasi Least Trimmed Square (LTS), Estimasi Scale (S), dan Estimasi Method of Moment (MM) pada Analisis Regresi Robust
Abstract
Abstract. Multiple linear regression is a statistical method that studies and measures the statistical relationship between two or more variables that contain causality in order to obtain a regression model. One method of estimating the parameters of the multiple linear regression model is the Ordinary Least Square. The method must pass several regression assumptions. The residual normality test is one of the regression assumptions that sometimes cannot be fulfilled. The reason is because there are outliers in the data. Therefore, to overcome these problems, a robust regression method is needed to overcome outliers. Robust regression is a method used when the distribution of the residuals is not normal and or there are some outliers that affect the model. The types of estimation methods found in robust regression are Least Trimmed Square (LTS) estimation, Scale (S) estimation, and Method Of Moment (MM) estimation. The purpose of this study is to obtain the best robust regression method resulting from the comparison of the LTS estimation method, S estimation, and MM estimation based on the smallest Residual Standard Error (RSE) value and largest adjusted R-square value. The data in this study is agricultural data for the Indonesian country in 2019. This data includes three variables, namely the agricultural product (Y), the agricultural land area (X1) and the amount of rainfall (X2). The results of this study indicate that the robust regression estimation of LTS has a smaller RSE value and a larger adjusted R-square value than the S estimation method and the MM estimation . LTS estimation is better in estimating regression parameters than the S estimation method and MMÂ estimation.
Keywords: Outlier, Robust regression, LTS-estimation, S-estimation, MM-estimation.
Abstrak. Regresi linear berganda merupakan salah satu metode statistika yang mempelajari dan mengukur hubungan statistik antara dua atau lebih variabel yang mengandung sebab akibat dengan tujuan untuk mendapatkan model regresi. Salah satu metode penduga parameter model regresi linier berganda adalah metode kuadrat terkecil (MKT). Metode tersebut harus memenuhi beberapa asumsi regresi. Uji normalitas residual salah satu asumsi regresi yang cukup sering tidak dapat terpenuhi. Alasan asumsi tersebut tidak dapat terpenuhi karena terdapat outlier pada data. Oleh karena itu, dalam mengatasi permasalahan tersebut diperlukan metode regresi robust untuk mengatasi pencilan. Regresi robust adalah metode yang digunakan ketika distribusi dari residual tidak normal dan atau adanya beberapa pencilan yang berpengaruh pada model. Beberapa metode estimasi yang terdapat pada regresi robust adalah estimasi Least Trimmed Square (LTS), estimasi Scale (S), dan estimasi Method Of Moment (MM). Adapun tujuan dari penelitian ini untuk menetukan metode regresi robust terbaik yang dihasilkan dari perbandingan metode estimasi LTS , estimasi S , dan estimasi MM berdasarkan nilai Residual Standard Error (RSE) terkecil dan nilai adjusted R-square terbesar. Data yang digunakan dalam penelitian ini adalah data pertanian dan curah hujan di Indonesia tahun 2019. Data ini meliputi tiga variabel yaitu variabel produksi padi (Y), luas panen (X1) dan curah hujan (X2). Hasil penelitian ini menunjukkan bahwa regresi robust estimasi LTS memiliki nilai RSE yang lebih kecil dan adjusted R-square yang lebih besar dibandingkan metode estimasi S maupun estimasi MM sehingga metode estimasi Least Trimmed Square (LTS) lebih baik dalam mengestimasi parameter regresi dibandingkan metode estimasi Scale (S) maupun estimasi Method Of Moment (MM).
Kata Kunci: Outlier, Regresi robust, estimasi LTS, estimasi S, estimasi MM.
Keywords
Full Text:
PDFReferences
Badan Pusat Statistik Indonesia. (2020). Statistik Indonesia 2020.
Chen, C. (2002). Robust Regression and Outlier Detection with ROBUSTREG Procedure. SAS Institute Inc. (Online). (https://pdfs.semanticscholar.org/ccb3/3dfc93f60ddb9f488533b8d85081c550a7d8.pd, diakses 20 Januari 2021)
Dehon, C., Gassner, M., & Verardi, V. (2008). A New Hausmann Type Test to Detect the Presence of Influential Outliers (No. 2008_006). ULB Universite Libre de Bruxelles.
Fox, J. & Weisberg, S. (2010). Robust Regression in R . Apendix to An R and S-Plus Companion to Applied Regression, Second Edition. (Online).(https://socserv.socsci.mcmaster.ca/jfox/Books/Companion/appendix/Appendix-RobustRegression.pdf, diakses 20 Januari 2021)
Maharani, I.F., Satyahadewi, N., & Kusnandar, D. (2014). Metode Ordinary Least Squares dan Least Trimmed Squares Dalam Mengestimasi Parameter Regresi Ketika Terdapat Outlier. Buletin Ilmiah Mat, Stat, dan Terapannya (Bimaster), Vol. 03, No. 3, 163-168.
Nurcahyadi, H. (2010). Analisis regresi pada data outlier dengan menggunakan Least Trimmed Square (LTS) dan MM-Estimasi.
Olive, D.J. (2005). Applied Robust Statistics. Carbondale: Southern Illinois University.
Rousseeuw, P.J., & Leroy, A.M. (1987). Robust Regression and Outlier Detection. New York: John Wiley and Sons.
Yohai, Victor J. (1987). High Breakdown Point and High Efficiency Robust Estimates For Regression. The Annals of Statistics, 642-656.
Sembiring, R.K. (1995). Analisis Regresi. Penerbit ITB. Bandung.
Soemartini. (2007). Pencilan (Outlier). Bandung: Universitas Padjajaran.
Utama Muhammad Bangkit Riksa, Hajarisman Nusar. (2021). Metode Pemilihan Variabel pada Model Regresi Poisson Menggunakan Metode Nordberg. Jurnal Riset Statistika, 1(1), 35-42.
DOI: http://dx.doi.org/10.29313/.v0i0.29248
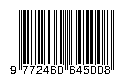