Penerapan Metode Extreme Learning Machine pada Peramalan Jumlah Penumpang Penerbangan Domestik di Kepulauan Riau
Abstract
Abstract. Extreme learning machine (ELM) is a new learning method from feedforward neural networks. The new learning of ELM is to overcome in terms of learning speed. The ELM method has a very fast learning speed compared to the old method algorithm which takes a very long time even for simple applications. In this research, ELM will be discussed to find out the forecasting method and prediction results for the number of passengers on domestic flights in the Kepulauan Riau. The results showed that with 8 hidden layers, 5 input data and 400 iterations to determine the optimal input weight and bias, the MSE value was 0.0226085. Forecasting results have the same pattern as the original data, but in April and May 2020 there are far predictions because there is a government regulation to close the airport from April 24 to June 1 to reduce the spread of COVID-19 but from July 2020 the prediction results begin to follow the pattern in the original data again.
Keywords: Extreme Learning Machine, Artificial Neural Network, and Feedforward.
Abstrak. Extreme learning machine (ELM) termasuk metode pembelajaran baru dari jaringan syaraf tiruan feedforward. Pembelajaran baru dari ELM adalah untuk mengatasi dalam hal kecepatan pembelajaran (learning speed). Metode ELM memiliki learning speed yang sangat cepat dibandingkan dengan algoritma metode lama yang membutuhkan waktu yang sangat lama walaupun hanya untuk aplikasi sederhana. Dalam penelitian ini akan dibahas mengenai ELM untuk mengetahui metode peramalan dan hasil prediksi untuk jumlah penumpang pada penerbangan domestik di Kepulauan Riau. Hasil penelitian menunjukkan bahwa dengan 8 hidden layer, 5 data input dan 400 iterasi untuk menentukan input weight dan bias yang optimal didapatkan nilai MSE sebesar 0.0226085. Hasil peramalan memiliki pola yang sama dengan data asli namun pada bulan April dan Mei tahun 2020 terdapat hasil prediksi yang cukup jauh karena terdapat aturan pemerintah untuk menutup bandar udara terhitung sejak tanggal 24 April hingga 1 Juni untuk menurunkan penyebaran COVID-19 tetapi mulai bulan Juli 2020 hasil prediksi mulai mengikuti lagi pola pada data asli.
Kata Kunci: Extreme Learning Machine, Jaringan Syaraf Tiruan, dan Feedforward.
Keywords
Full Text:
PDFReferences
Alfiyatin, A. N., Rizki, A. M., Ananda, C. F., & Mahmudy, W. F. (2019). Extreme Learning Machine and Particle Swarm Optimization for Inflation Forecasting. (IJACSA) International Journal of Advanced Computer Science and Applications, 473-478.
Ginting, A. M. (2013). Kendala Pembangunan Provinsi Daerah Kepulauan: Studi Kasus Provinsi Kepulauan Riau. Politica, 49-75.
Giusti, A., Widodo, A. W., & Adinugroho, S. (2018). Prediksi Penjualan Mi Menggunakan Metode Extreme Learning Machine (ELM) di Kober Mie Setan Cabang Soekarno Hatta. Jurnal Pengembangan Teknologi Informasi dan Ilmu Komputer Vol.2, No. 8, 2972-2978.
Huang, G. B., Zhu, Q. Y., & Siew, C. K. (2004). Extreme Learning Machine: A New Learning Scheme of Feedforward Neural Networks. International Joint Conference on Neural Networks (IJCNN2004), 25-29.
Huang, G. B., Zhu, Q. Y., & Siew, C. K. (2006). Extreme Learning Machine: theory and applications. Neurocomputing, 70(1), pp.489-501.
Jain, Y. K., & Bhandare, S. K. (2011). Min max normalization based data perturbation method for privacy protection. International Journal of Computer & Communication Technology, 2(8), pp.45-50.
Kennedy, J., & Eberhart, R. (1995). Particle Swarm Optimization. Proceeding of IEEE International Conference on Neural Networks, Vol.6.
Kusumadewi, S. (2003). Artificial Intelligence (Teknik dan Aplikasinya). Yogjakarta: Graha Ilmu.
Makridakis, S., & Hibon, M. (1995). Evaluating Accuracy (Or Error) Measures. Volume 9518 of Working papers/INSEAD.
Sateria, A., Saputra, I. D., & Dharta, Y. (2018). Penggunaan Metode Particle Swarm Optimization (PSO) pada Optimasi Multirespon Gaya Tekan dan Momen Torsi Penggurdian Material Komposit Glass Fiber Reinforce Polymer (GFRP) yang Ditumpuk dengan Material Stainless Steel (SS). JURNAL MANUTECH Vol.10, No.1, Juni 2018: 2- 57 .
Setiani, B. (2015). Prinsip-Prinsip Pokok Pengelolaan Jasa Transportasi Udara. Jurnal Ilmiah WIDYA, 103-109.
Yulianto Anggi Priliani, Darwis Sutawanir. (2021). Penerapan Metode K-Nearest Neighbors (kNN) pada Bearing. Jurnal Riset Statistika, 1(1), 10-18.
DOI: http://dx.doi.org/10.29313/.v0i0.29177
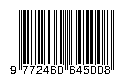