Penerapan Metode Generalized Space Time Autoregressive Model terhadap Penderita Penyakit Demam Berdarah Dengue (DBD)
Abstract
Abstract. Generalized Space Time Autoregressive (GSTAR) model, is one of the forecasting methods for time series modeling that contains time and location elements with autoregressive order (p) and spatial order ( ). This study will discuss the application of the GSTAR model using three different location weights, namely uniform location weight, distance inverse location weight, and cross-correlation normalization location weights on the data on the number of patients with Dengue Hemorrhagic Fever (DHF) in four districts of Bandung City. From the GSTAR model that is formed, the best model will be selected from the three location weights based on the smallest Root Mean Square Error (RMSE) value. The results of this study by applying the data on the number of dengue patients obtained by the GSTAR ((11) - (I1)) model with a weighted distance inverse location which has an RMSE value of 0.87441 smaller than the GSTAR model ((11) - (I1)) with other location weighters. The model used is the GSTAR ((11) - (I1)) model with a weighted inverse distance.
Keywords: Model GSTAR, Autoregressive, orde Spasial,forecasting,RMSE, DHF
Abstrak. Generalized Space Time Autoregressive (GSTAR) model, merupakan salah satu metode peramalan untuk pemodelan deret waktu yang mengandung unsur lokasi dan waktu dengan orde autoregressive (p) dan orde spasial ( ). Pada penelitian ini akan dibahas mengenai penerapan model GSTAR dengan menggunakan tiga bobot lokasi yang berbeda yaitu bobot lokasi seragam, bobot lokasi invers jarak, dan bobot lokasi normalisasi korelasi silang pada data jumlah penderita penyakit Demam Berdarah Dengue (DBD) di empat Kecamatan Kota Bandung. Dari model GSTAR yang terbentuk akan dipilih model terbaik dari ketiga bobot lokasi berdasarkan nilai Root Mean Square Error (RMSE) terkecil. Hasil penelitian ini dengan menerapkan data jumlah penderita penyakit DBD diperoleh model GSTAR ((11)-(I1)) dengan pembobot lokasi invers jarak yang memiliki nilai RMSE sebesar 0,87441 lebih kecil dari pada model GSTAR ((11)-(I1)) dengan pembobot lokasi lainnya. Model yang digunakan yaitu model GSTAR ((11)-(I1)) dengan pembobot lokasi invers jarak.
Kata Kunci: Model GSTAR, Autoregressive, orde Spasial, peramalan,RMSE, DBD
Keywords
Full Text:
PDFReferences
Caraka, R. E., Sugiyarto, W., Erda, G., & Sadewo, E. (2016). Pengaruh Iflasi Terhadap Impor dan Ekspor Di Provinsi Riau dan Kepulauan Riau menggunakan Generalized Spatio Time Series. Badan Pendidikan dan Pelatihan Keuangan Kementrian Keuangan Republik Indonesia Vol.9 n0.1, 180-198.
Islamiyah , A. N., Rahayu, W., & Wiraningsih, E. D. (2018). Pemodelan Generalized Space Time Autoregressive (GSTAR) dan Penerapannya pada Penderita TB Paru (BTA+) di DKI Jakarta. Jurnal Statistika dan Aplikasinya Vol.2 No.2, 36-48.
Nurmalasri, R., D. I. (2017). Analisis Faktor-Faktor Yang Mempengaruhi Indeks Pembangunan Manusia (IPM) Menggunakan Metode Regresi Logistik Ordinal Dan Regresi Probit Ordinal. GAUSIAN, 111-120.
Handayani, R., S. W. (2018). Pemodelan Generalize Space Time Autoregressive (GSTAR) Pada Data Inflasi di Kota Samarinda. Eksponensial Volume 9, 153-162.
S.Stay, R. (2014). Multivariate Time Series Analysis With R and Financial Application. Canada: Wiley Interscience.
Wei, W. W. (2019). Multivariate Time Series Analysis and Applications. Wiley.
Yanti, T. S. (2009). Model Pengganda Uang untuk Menentukan Jumlah Uang Beredar di Indonesia Menggunakan Model ARIMA Komponen. Statistika Vol.9 No.1, 25-32.
DOI: http://dx.doi.org/10.29313/.v6i2.23003
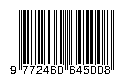